North Texas’ resident tech genius, John Carmack, is taking aim now at his most ambitious target: solving the world’s biggest computer-science problem by developing artificial general intelligence. That’s a form of AI whose machines can understand, learn, and perform any intellectual task that humans can do.
Inside his multimillion-dollar manse on Highland Park’s Beverly Drive, Carmack, 52, is working to achieve AGI through his startup Keen Technologies, which raised $20 million in a financing round in August from investors including Austin-based Capital Factory.
This is the “fourth major phase” of his career, Carmack says, following stints in computers and pioneering video games with Mesquite’s id Software (founded in 1991), suborbital space rocketry at Mesquite-based Armadillo Aerospace (2000-2013), and virtual reality with Oculus VR, which Facebook (now Meta) acquired for $2 billion in 2014. Carmack stepped away from Oculus’ CTO role in late 2019 to become consulting CTO for the VR venture, proclaiming his intention to focus on AGI. He left Meta in December to concentrate full-time on Keen.
We sat down with the tech icon during a rare break in his work to conduct the following exclusive interview—a frank conversation that took Dallas Innovates weeks to arrange. The Q&A has been edited for length and clarity.
What sort of work are you doing now to ‘solve’ artificial general intelligence, John, and why are you taking your particular approach?
I sit here at my computer all the time, thinking up concepts, documenting them, making theories, testing them. That’s the work right now, as nobody really knows the full path all the way to where we want to go. But I think I’ve got as good a shot at it as anyone, for a number of reasons.
“Some people have raised billions to pursue this. And while that’s interesting in some ways, and there are signs there are extremely powerful things possible now in the narrow machine-learning stuff, it’s not clear those are the necessary steps to get all the way to artificial general intelligence.”
Some people have raised billions of dollars to pursue this. And while that’s interesting in some ways, and there are signs that extremely powerful things are possible right now in the narrow machine-learning stuff, it’s not clear that those are the necessary steps to get all the way to artificial general intelligence. For companies that are happy to do that, it’s not a bad bet, because there are plenty of off-ramps where there are valuable things, even if you don’t get all the way. There’s still stuff that’s going to change the world, like the narrow AI.
But it’s a worry that if you just take the first off-ramp and say, ‘Hey, there’s a billion-dollar off-ramp right here’—where we know we can just go take what we understand and revolutionize various industries. That becomes a very tempting thing to do, but it distracts everyone from looking further ahead and focusing on the big far distance stuff. So, I’m in a position where I can be really blunt about what I’m doing, and that is: zero near-term business opportunities.
What compelled your interest in the topic in the first place?
We’re in the midst of a scientific revolution right now, because 10 years ago, there was not the sense that AI was working. We’ve had these AI ‘winters’—a couple of them over the decades, in fact. It’s funny, because virtual reality also went through this: It had almost been a bad word because it had crashed so bad in the 1990s, people didn’t even want to talk about it.
And artificial intelligence had a couple of those cycles where hype spins up, money flows in, it underperforms, and then it crashes, and nobody wants to talk about it. But this last decade was different, and people who don’t notice how different it is this time aren’t paying attention, because the number of absolutely astounding things that have happened in the last decade with machine learning is really profound.
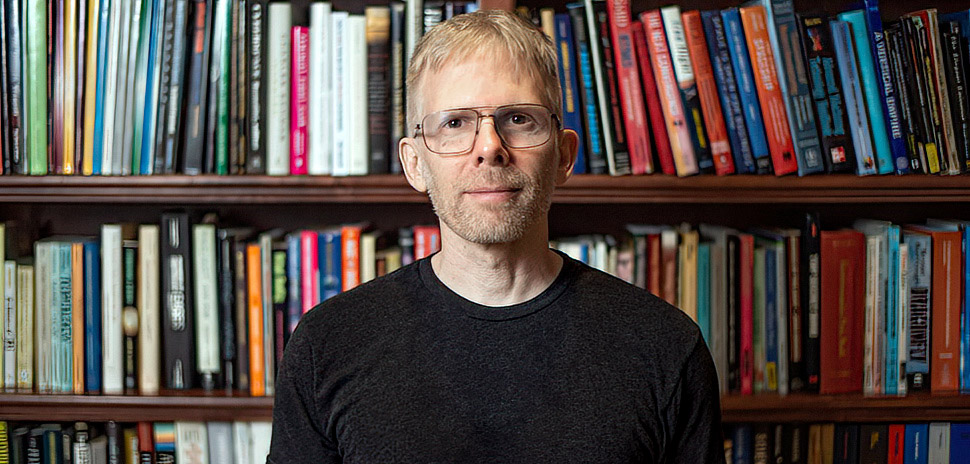
[Photo of John Carmack by Michael Samples]
That was the thing that really pushed me toward saying, ‘All right, it’s probably time to take a serious look at this.’ And it was interesting for me, because I had a technical bystander’s understanding of machine learning and AI, where I had read some of the seminal books even in my teenage years, and I knew about symbolic AI and all those types of things. So, my brain knew a little bit about these things, but I wasn’t following what was going on because I was busy with my work with the games, the aerospace, and the virtual reality.
You get to that point where you recognize, ‘Okay, I think there’s probably something here I need to sort out—what is hype versus what is the reality?’ So I did what I usually do: All of my real abilities have always come from understanding things fundamentally, at the very deepest levels, where there are insights that you only get from knowing how things happen from the very bottom.
So, about four years ago, I went on one of my week-long retreats, where I just take a computer and a stack of reference materials and I spend a week kind of reimplementing the fundamentals of the industry. And getting to the point where it’s like, ‘All right, I understand this well enough to have a serious conversation with a researcher about it.’ And I was pretty excited about getting to that level of understanding.
“So I asked Ilya Sutskever, OpenAI’s chief scientist, for a reading list. He gave me a list of like 40 research papers and said, ‘If you really learn all of these, you’ll know 90% of what matters today.’ And I did. I plowed through all those things and it all started sorting out in my head.”
Then after that, Sam Altman of OpenAI invited me to a conference—the Y Combinator’s YC 120—and, while historically I never go to these types of things (because of my hermit tendencies), this time I decided to attend. It turned out it was really orchestrated by Sam to jump me for a job pitch, because he had Greg Brockman and Ilya Sutskever of OpenAI come and try to get me to go to OpenAI. I was pretty flattered by that, because I was not a machine learning expert by any means. I was a well-known systems engineer on a lot of this stuff, but I only had this basic baseline knowledge [of AI]. So, the idea that they were the leaders in the field, and they thought I was worth trying to get there, that really planted the seed to make me think about the importance of everything that’s going on and what role I could play in it.
So I asked Ilya, their chief scientist, for a reading list. This is my path, my way of doing things: give me a stack of all the stuff I need to know to actually be relevant in this space. And he gave me a list of like 40 research papers and said, ‘If you really learn all of these, you’ll know 90% of what matters today.’ And I did. I plowed through all those things and it all started sorting out in my head.
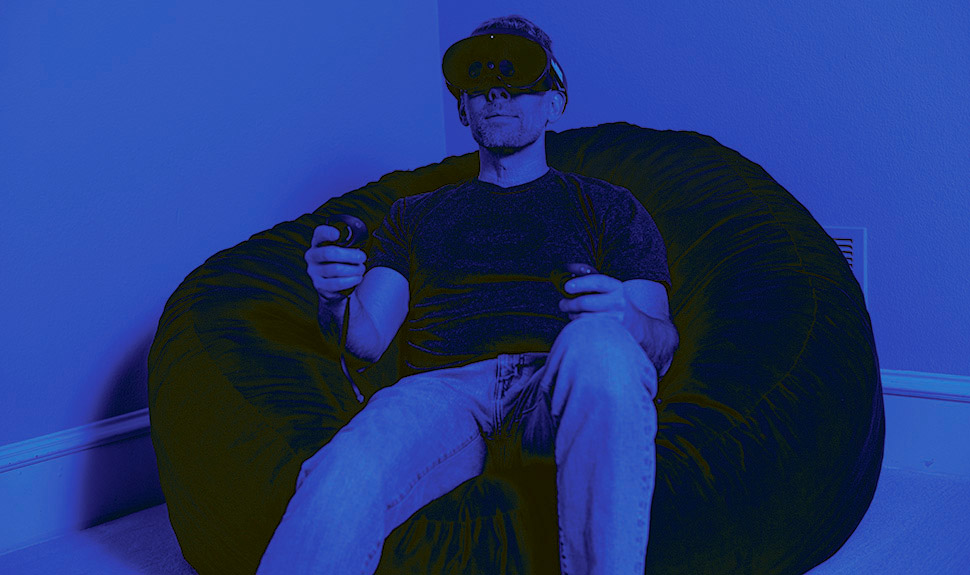
John Carmack in his VR room. [Photo: Michael Samples]
You were still with Meta at this time working on virtual reality, right?
Yes, and I was having some real issues at Meta with large-scale strategic directions. I’m sure you’ve seen some of the headlines about how much money they’re spending, and I thought large fractions were really poorly spent. I was having some challenges there, and I was at the end of my five-year buyout contract from when Oculus was acquired. That was when I decided, ‘Okay, I’m going to get more serious about this artificial general intelligence work.’
All the things that I’d done before—in games, rockets, virtual reality—I was aiming for something that wasn’t there yet, but I had a clear line of sight. It’s different for AGI, though, because nobody knows how to do it. It’s not a simple matter of engineering. But there are all these tantalizing clues given what happened in this last decade—it’s like a handful of relatively simple ideas. They’re not these extreme black-magic mathematical wizardries—a lot of them are relatively simple techniques that make perfect sense to me now that I understand them. And it feels like we are a half-dozen more insights away from having the equivalent of our biological agents.
I made an estimate three or four years ago that I think there’s a 50-50 chance that we’ll have clear signs of life in 2030 of artificial general intelligence. That doesn’t necessarily mean a huge economic impact for anything yet, but just that we have a being that’s running on computers that most people recognize as intelligent and conscious and sort of on the same level of what we humans are doing. And after three years of hardcore research on all this, I haven’t changed my prediction. In fact, I probably even slightly bumped it up to maybe a 60% chance in 2030. And if you go up to, say, 2050, I’ve got it at like a 95% chance.
Many are predicting stupendous, earth-shattering things will result from this, right?
I’m trying not to use the kind of hyperbole of really grand pronouncements, because I am a nuts-and-bolts person. Even with the rocketry stuff, I wasn’t talking about colonizing Mars, I was talking about which bolts I’m using to hold things together. So, I don’t want to do a TED talk going on and on about all the things that might be possible with plausibly cost-effective artificial general intelligence.
But especially the pandemic showed that more things than people thought could be done strictly through a computer interaction stream, where you can communicate over the computer modalities like Zoom, email, chats, Discord, all these things.
A large fraction of the world’s value today can run on that. And if you have an artificial agent that behaves like a human being—even in our narrow AIs today, the world of deep fakes and chat bots and voice synthesis—it’s clear that we can simulate the human modalities there. We do not yet have the learnable stream of consciousnesses of a co-worker in AI, but we do have kind of this oracular amount of knowledge that can be brought forward.
You’ll find people who can wax rhapsodic about the singularity and how everything is going to change with AGI. But if I just look at it and say, if 10 years from now, we have ‘universal remote employees’ that are artificial general intelligences, run on clouds, and people can just dial up and say, ‘I want five Franks today and 10 Amys, and we’re going to deploy them on these jobs,’ and you could just spin up like you can cloud-access computing resources, if you could cloud-access essentially artificial human resources for things like that—that’s the most prosaic, mundane, most banal use of something like this.
If all we’re doing is making more human-level capital and applying it to the things that we’re already doing today, while you could say, ‘I want to make a movie or a comic book or something like that, give me the team that I need to go do that,’ and then run it on the cloud—that’s kind of my vision for it.
Why is it so important to achieve a system that performs tasks that humans can do? What’s wrong with humans doing human tasks?
Well, you can tie that into a lot of questions, like, ‘Is human population a good thing?’ ‘Is immigration a good thing, where we seem to have been able to take advantage of new sources of humanity that are willing to engage in economic activities and be directed by the markets?’
The world is a hugely better place with our 8 billion people than it was when there were 50 million people kind of like living in caves and whatever. So, I am confident that the sum total of value and progress in humanity will accelerate extraordinarily with welcoming artificial beings into our community of working on things. I think there will be enormous value created from all that.
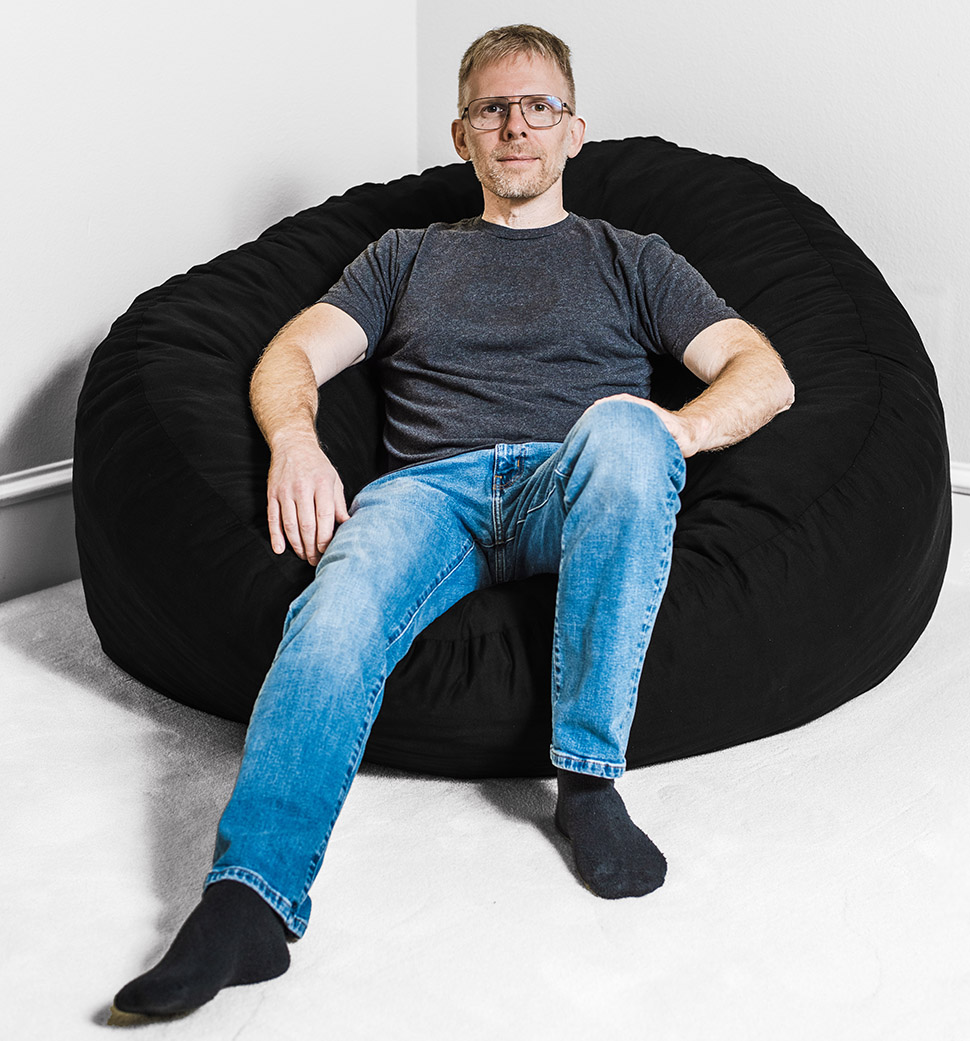
[Photo of John Carmack by Michael Samples]
So, how do you see the particular route that will get to achieving AGI?
There’s a path that leads from today’s virtual assistants—your Siris, Alexas, and Google Assistants—to being more and more helpful, taking over more and more tasks. But those are fairly brittle, specialized implementations of things—various knowledge representations, voice synthesis, voice understanding—and that’s probably not the path to a general intelligence that’s flexible for a whole bunch of purposes. They have thousands of programmers, literally, working right now on adding capabilities to those assistants, and there is near-term value in that. The programming work that’s done to stitch those things together is going to be throw-away programming. But that path doesn’t lead to the general agent that can learn any task that a human can.
The things dealing with perception—like understanding someone’s voice, and even synthesizing voices naturally—those were the things that computers did not do well at all 10 or 15 years ago. The joke in the ‘90s was that you had a computer that could beat the world chess champion handily, but a computer couldn’t do things that a 2-year-old could do: It couldn’t tell a cat from a dog. There was no computer product in the world that could do the simple trivial perception things. Because, it turned out, that’s what our brain actually does: It’s much more about perception and pattern matching. And it was sort of the sophistry of the people then to think instead that it’s about these philosophical symbol manipulation things. And that led AI astray, really, for decades.
There were all these really blind alleys that turned out to be fragile things and not very commercially valuable. It just wasn’t the way things worked. But then came the revolution of this last decade: With deep learning and the deep connectionist approaches, we actually can do all those things that the 2-year-olds can do in terms of perception. And in many of these, we’re at a superhuman level. The thing we don’t yet have is sort of the consciousness, the associative memory, the things that have a life and goals and planning. And there are these brittle, fragile AI systems that can implement any one of those things, but it’s still not the way the human brain or even the animal brain works. I mean, forget human brains; we don’t even have things that can act like a mouse or a cat. But it feels like we are within striking distance of all those things.
I think that, almost certainly, the tools that we’ve got from deep learning in this last decade—we’ll be able to ride those to artificial general intelligence. There are some of the structural things that we don’t understand yet about these other fields, like you have reinforcement learning, supervised learning, unsupervised learning. All of those come together in the way humans think about things, and we don’t have the final synthesis of all that yet.
Is there a critical factor or central idea for getting there?
One of the things I say—and some people don’t like it—is that the source code, the computer programming necessary for artificial general intelligence, is going to be a few tens of thousands of lines of code. Now, a big program is millions of lines of code—the Chrome browser is like 20 to 30 million lines of code.
Elon just mentioned that Twitter runs on like 20 million lines of Scala. These are big programs, and there’s no chance that one person can go and rewrite that. You literally cannot type enough in your remaining life to write all of that code. But it’s my belief that I can really back up that the programming for AGI is going to be something that one person could write.
Now, the smart money still says it’s done by a team of researchers, and it’s cobbled together over all that. But my reasoning on this is: If you take your entire DNA, it’s less than a gigabyte of information. So even your entire human body is not all that much in the instructions, and the brain is this tiny slice of it —like 40 megabytes, and it’s not tightly coded. So, we have our existence proof of humanity: What makes our brain, what makes our intelligence, is not all that much code.
Now, it evolves into an extraordinarily complex object, and the numbers that you’ll see tossed around are that the human brain has about 86 billion neurons and maybe up to 100 trillion connections between them. Now, even in computer terms, that’s a big number. When you talk about the big models, like GPT-3 or whatever, you say, ‘Oh, it’s 160 billion parameters’—the parameters being sort of similar to the connections in the brain.
So, you might say that we still have a factor of 500 more or so to go before our computers have as much capability as the brain. But I think there’s also a very good reason to believe that that is an extremely pessimistic estimate, that the estimate should be much smaller, because our brains are doing lots of things that aren’t really that important. They’re really sloppy, they’re really slow, so they probably do not need that many parameters.
But again, it’s a simple program exploited at massive scale, which is exactly what’s happening with the AIs of today. If you took the things that people talk about—GPT-3, Imagen, AlphaFold—the source code for all these in their frameworks is not big. It’s thousands of lines of code, not even tens of thousands. Now, they’re built on top of a big framework of supporting ecosystem stuff, but the core logic is not a big program.
So, I strongly believe that we are within a decade of having reasonably commonly available sufficient hardware for doing this, that it’s going to be a modest amount of code, and that there are enough people working on it. Although in my mind, it’s kind of surprising that there aren’t more people in my position doing it, while everybody looks at DeepMind and OpenAI as the leading AGI research labs.
Why do you want to work independently of all these people?
The reason I’m staying independent is that there is this really surprising ‘groupthink’ going on with all the major players. It’s been almost bizarre in the last year to see things like: OpenAI releases an image generator, then Google releases one, then Facebook releases one. So, all of these companies are just within a couple of months of being able to reproduce anybody else’s work, because they all draw from the same academic researcher pool. There’s cross-pollination and an enormous brain trust of super-brilliant people doing all this.
“While I’ve got people that invested $20 million in my company, I’m not telling them that I’m likely to have the breakthrough for artificial general intelligence. Instead, I’m saying there’s a non-negligible chance that I will personally figure out some of the important things that are necessary for this.”
But, because we don’t know where we’re going yet, there is actually a strategy inside machine learning where you need a degree of randomness—where you start with random weights and random locations and sometimes multiple ensemble models. So, I am positioning myself as one of these random test points, where the rest of the industry is going in a direction that’s leading to fabulous places, and they’re doing a great job on that. But, because we do not have that line of sight—we’re not sure that we’re in the local attractor basin where we can just gradient descent down to the solution for this—it’s important to have some people testing other parts of the solution space as well.
And, I have a different background. I’m not from an academic research background—I’m a systems engineer. I’ve got some of these perceptions and systems technology and emergent behavior pieces that are relevant to this, and I’m smart enough to apply the necessary things. So, while I’ve got people that invested $20 million in my company, I’m not telling them that I’m likely to have the breakthrough for artificial general intelligence. Instead, I’m saying there’s a non-negligible chance that I will personally figure out some of the important things that are necessary for this.
Once it’s figured out, what do you think the ramifications will be?
The emergence of artificial general intelligence in a way that can impact the economy really is a ‘change-the-world-level’ event, where this is something that reshapes almost everything that human beings can do. This is something that is almost the largest scale that you can think about. So, it’s worth some of these bets, like the $20 million on my research directions. It might pan out, it probably won’t. I can say that just flat-out. No, the odds that I will come up with this before everybody working at OpenAI and DeepMind and all the Chinese research labs—it would be incredibly hubristic to say, ‘Yes, I’m confident I’m going to get there first.’
But, I’m not aware of anyone that I think is significantly smarter than I am working on these problems. I think that I am not out of my league playing in this game. And I am taking a different path. I’m in a position where I can say, ‘Yes, I’m going to throw the next decade of my life at this, and it might be this grand spectacular success.’ Or, it could turn out I find two super-clever things and I partner up with somebody else. Maybe there’s an acquisition or something down the road there.
But what I don’t want to do is pick the very first commercial application and say, ‘Okay. I know gaming, I know image generation, I could go do gaming content creation.’ And in fact, my ex-partner from Oculus, Brendan Iribe, was saying, ‘Come do this with me. We’re going to raise a bunch of money, it’ll be great.’ And yes, that’s an almost guaranteed unicorn. And there’s very little doubt we could spin up a billion-dollar company doing that. But the big brass ring—artificial general intelligence—that’s trillions. It’s different orders of magnitude.
I’m lucky enough to be in this position where I’ve got my successes, I’ve got my achievements, I’ve got my financial stability. So I can take this bet and take this risk, and it is extremely risky. But because I’m not worried about ruin, I can say, ‘Okay, if I think I’ve got a couple percent chance of doing this, and it’s worth trillions, that’s not a bad bet.’ I mean, that’s a bad way for most people to think, but I’m in a situation where it’s not a bad thing.
So, how exactly are you placing this ‘bet’ at Keen right now?
It’s research and development, where I’ve got a handful of ideas that are not the mainstream. I follow most of what the mainstream is doing because it’s fabulous, it’s useful. Right now I’m following up on some research papers from last year that I think have more utility for the way I want to apply them than what their original authors were looking at.
There’s valuable things that happened earlier that people aren’t necessarily aware of. There’s some work from like the ’70s, ’80s, and ’90s that I actually think might be interesting, because a lot of things happened back then that didn’t pan out, just because they didn’t have enough scale. They were trying to do this on one-megahertz computers, not clusters of GPUs.
And there is this kind of groupthink I mentioned that is really clear, if you look at it, about all these brilliant researchers—they all have similar backgrounds, and they’re all kind of swimming in the same direction. So, there’s a few of these old things back there that I think may be useful. So right now, I’m building experiments, I’m testing things, I’m trying to marry together some of these fields that are distinct, that have what I feel are pieces of the AGI algorithm.
But most of what I do is I run simulations through watching lots of television and playing various video games. And I think that combination of, ‘Here’s how you perceive and internalize a model of the world, and here’s how you act in it with agency in some of these situations,’ I still don’t know how they come together. But I think there are keys there. I think I have my arms around the scope of the problems that need to be solved, and how to push things together.
I still think there’s a half dozen insights that need to happen, but I’ve got a couple of things that are plausible insights that might turn out to be relevant. And one of the things that I trained myself to do a few decades ago is pulling ideas out and pursuing them in a way where I’m excited about them, knowing that most of them don’t pan out in the end. Much earlier in my career, when I’d have a really bright idea that didn’t work out, I was crushed afterwards. But eventually I got to the point where I’m really good at just shoveling ideas through my processing and shooting them down, almost making it a game to say, ‘How quickly can I bust my own idea, rather than protecting it as a pet idea?’
So, I’ve got a few of these candidates right now that I’m in the process of exploring and attacking. But it’s going to be these abstract ideas and techniques and ways to apply things that are similar to the way deep learning is done right now.
So, I’m pushing off scaling it out, because there are a bunch of companies now saying, ‘We need to go raise $100 million, $200 million, because we need to have a warehouse full of GPUs.’ And that’s one path to value, and there’s a little bit of a push toward that. But I’m very much pushing toward saying, ‘No, I want to figure out these six important things before I go waste $100 million of someone’s money.’ I’m actually not spending much money right now. I raised $20 million, but I’m thinking that this is a decade-long task where I don’t want to burn through $20 million in the next two years, then raise another series to get another couple hundred million dollars, because I don’t actually think that’s the smart way to go about things.
“My hope is that I can spend several years working through some of these things, building small things that I think point in the right directions. And then, throw some scale at it and push an entire lifetime of information and experience through this and see if it comes out with something that shows that spark.”
My hope is that I can spend several years working through some of these things, building small things that I think point in the right directions. And then, throw some scale at it and push an entire lifetime of information and experience through this and see if it comes out with something that shows that spark. Because again, I don’t expect how to pop out of this.
What I keep saying is that as soon as you’re at the point where you have the equivalent of a toddler—something that is a being, it’s conscious, it’s not Einstein, it can’t even do multiplication—if you’ve got a creature that can learn, you can interact with and teach it things on some level. And at that point you can deploy an army of engineers, developmental psychologists, and scientists to study things.
Because we don’t have that yet, we don’t have the ability to simulate something that’s a being like that. There are tricks and techniques and strategies that the brain is doing that none of our existing models do. But getting to that point doesn’t look out of reach to me.
Can you see yet how to arrive at that out-of-reach point?
I see the destination. I know it’s there, but no, it’s murky and cloudy in between here and there. Nobody knows how to get there. But I’m looking at that path saying I don’t know what’s in there, but I think I can get through there—or at least I think somebody will. And I think it’s very likely that this is going to happen in the 2030s.
I do consider it essentially inevitable. But so much of what I’ve been good at is bringing something that might be inevitable forward in time. I feel like the 3D video gaming stuff that I did, it probably always would have happened, but it would have happened years later if I hadn’t made it happen earlier.
Quincy Preston contributed to this report.
A version of this story was originally published in Dallas Innovates 2023.
Read Dallas Innovates 2023 online
Take a journey into the heart of North Texas business. Our annual magazine takes you on a tour of the innovative and creative forces shaping the future.
WHAT ARE YOU INNOVATING? Let us know.
Get on the list.
Dallas Innovates, every day.
Sign up to keep your eye on what’s new and next in Dallas-Fort Worth, every day.